The opportunities within GenAI for a business are astoundingly limitless. Able to support interactions with customers, generate creative content for marketing and sales, and draft computer code based on natural-language prompts, GenAI’s impact on productivity could add trillions of dollars in value to the global economy.1. Complex tools such as data augmentation and synthetic data generation can be used to test customer models in cases where privacy concerns are especially paramount.
Data quality lays at the foundation of any GenAI initiative, driving sustainable success. Opportunities in customer research to improve R&D simulation and testing quality are only accessible when standards in governance, relevancy, and consistency are established. For AI systems to deliver meaningful and trustworthy outcomes, they must be fed with data that is both relevant and reliable.
Challenges when implementing GenAI without Data Quality
Putting data quality first is crucial when it comes to using GenAI to drive revenue. Clean and accurate data is the foundation on which AI models are built, ensuring unbiased and reliable outcomes.
As businesses rapidly adopt Generative AI, experts warn that neglecting data governance and quality measures can seriously limit the potential of GenAI and expose the organization to security and ethical risks.
- Addressing Potential Bias: One of the key concerns with AI models, especially in generative AI, is the presence of biases. Biased data can lead to biased AI model outputs, impacting the accuracy and fairness of the generated content. These biases often result from biased or inadequate data used to train the model; by applying data quality best practices, businesses can mitigate them.
- Data Integration and Centralization: Integrating data from various sources into a centralized repository is often an integral first step when implementing an AI initiative. The goal is to ensure centralized data and provide AI models with a single source of truth.
- Data classification: Effectively categorizing data can empower governance and security teams to implement the right controls but successful data governance depends upon differentiating sensitive, regulated information from data clutter – and the amount of unstructured data most enterprises manage can make this task near impossible if started too late in transformation efforts.
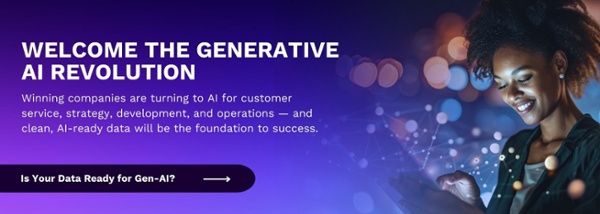
Steps Toward Data Quality for Gen AI Success
A Data First approach should be a priority for any business transformation, and Generative AI is no exception. To reduce project risk, start data cleansing and simulation from the onset. Improving data quality is the first step to fully leveraging the potential of generative AI technology. Best practices provide foundational steps that companies can take today to address data quality concerns and bring the business into the next generation of AI technologies.
- Data Governance: Data governance plays a crucial role in fostering innovation in GenAI within the organization by ensuring responsible data practices, mitigating biases, and safeguarding privacy. When used effectively, data governance and GenAI can drive compliance and privacy standards even in the most stringent regulatory environments.
- Data Cleansing & Validation: Regularly clean and preprocess data to eliminate errors, duplications, and inconsistencies, maintaining a high standard of data quality.
- Data Profiling & Quality Metrics: Implement data profiling tools to analyze and assess the quality of data with metrics to help measure completeness, accuracy, consistency, and timeliness, providing a quantitative measure of data reliability.
Data Quality: Laying the Groundwork for Generative AI
GenAI tools are only as good as the data they have access to. Without data quality and data governance capabilities, the potential impact and value added by Generative AI will be severely limited and may even expose organizations to data and cybersecurity risks. Use Data quality strategies to accelerate your GenAI implementation
The significance of putting data quality first in GenAI initiatives cannot be overstated, as it forms the bedrock for unbiased and reliable outcomes. Neglecting data governance and quality measures during GenAI implementation poses serious risks, ranging from compromised security to ethical concerns.
To navigate these challenges and unlock the full capabilities of Generative AI, businesses must prioritize data quality through governance, cleansing, validation, profiling, and the implementation of quality metrics. As GenAI continues to reshape industries, laying a robust data quality groundwork emerges as the essential precursor for sustainable success and innovation in the next generation of AI technologies.
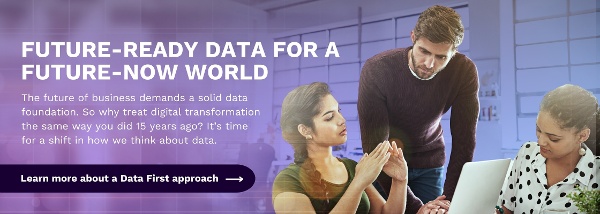
- McKinsey, The economic potential of generative AI: The next productivity frontier, June 14, 2023: https://www.mckinsey.com/capabilities/mckinsey-digital/our-insights/the-economic-potential-of-generative-ai-the-next-productivity-frontier#key-insights